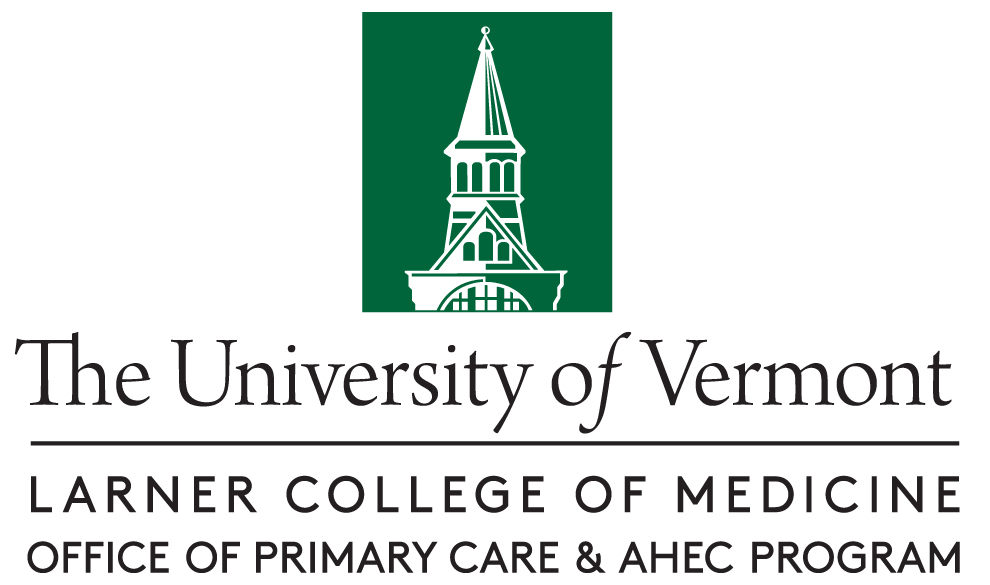
UVM AHEC
Document Type
Poster
Publication Date
2024
Focus Area
Medical Practice Transformation
Abstract
Context: UVM Health Network carried out a Pilot Study wherein 50 providers utilized 2 different Artificial Intelligence (AI) generating draft note programs (Abridge, Vendor X) over a 2-month period. Group A used Vendor X during the 1st month and Abridge during the 2nd month, while Group B used Abridge during the 1st month and Vendor X during the 2nd month.
Objective: This study aims to determine the quality of AI-generated drafted and edited notes in comparison to Provider only generated notes within UVMHN. Additionally, we want to determine if there is difference in note quality between the 2 AI note programs.
Study Design: To carry out our study we Identified the providers enrolled in the pilot study then established inclusion (AI-generated out-patient notes produced during the 2nd two-week period of each month) and exclusion criteria (we excluded physical exams, wellness visits, pre-op visits, tele-video visits, and well-child check visits).
Dataset: All notes that met that criteria were entered into an Excel database and classified according to: physician name, UVMHN site, clinical FTE, AI program used, and type of note (acute-care or follow-up). Based upon feedback from providers enrolled in the study we decided to focus our primary note quality analysis on Abridge drafted then edited notes versus Physician only generated notes and our secondary analysis on Abridge versus Vendor X. We determined that to adequately power our analysis based on previously seen differences in the literature, we required 46 of each note type for our primary analysis and 23 of each note type for our secondary analysis. We then randomly selected a proportion of AI-generated drafted and then edited notes from each potential note category (pilot study group, provider, type of note), such that we had a representative sample of notes to meet our established “N” number. For each Abridge generated drafted and edited note selected for primary analysis we identified a provider only generated note from the 6 months prior to the Pilot Study produced by the same provider and matched the note-type (we attempted to match the patient if available).
Population Studied: We analyzed AI generated and edited notes and physician only generated notes produced during patient visits with providers enrolled in the Pilot Study.
Instrument: Based on prior work in the literature that focused on the rating of AI-generated notes, we developed an 11-category rating tool utilizing a 5-point Likert scale.
Outcome Measures: We used 2 human raters to rate all notes included in the study. Each rater underwent a training session to familiarize themselves with the rating tool and each completed a 10-note training set with results included in final analysis. The notes included in the study were then rated and scores for each note averaged. The data was analyzed using paired t-tests. We then compared AI-generated note scores versus Provider-generated note scores to determine if there is a difference in note quality.
Results: Our results showed the Abridge generated then edited notes had an average score of 50 and provider only generated notes had a score of 48.6. Statistically there was no difference between these scores (p-value of 0.16). A difference in quality between Abridge-generated drafted and edited notes and Vendor "X"-generated drafted and edited notes was detected; specifically, that Abridge-generated note were of better quality than Vendor "X"-generated notes (p-value 0.0008).
Conclusions: Abridge AI generated drafted and edited notes were non-inferior to provider only generated notes. Using generative AI as a wellbeing intervention does not appear to impact quality of notes, however future work includes identifying if this quality extends to all note types and all specialty documentation. It was also concluded that not all AI vendor draft notes are of the same quality.
Creative Commons License
This work is licensed under a Creative Commons Attribution 4.0 International License.
Recommended Citation
Harrington, Edward; Cordero, Francisco; Cangiano, Michelle MD; and Jacobs, Alicia MD, "Comparison of Provider-Generated vs Artificial Intelligence-Generated Medical Encounter Notes" (2024). UVM AHEC. 13.
https://scholarworks.uvm.edu/uvmahec/13
Comments
Poster presented by Edward Harrington at The North American Primary Care Research Group (NAPCRG) annual meeting on Nov 20, 2024 in Quebec City, Canada.